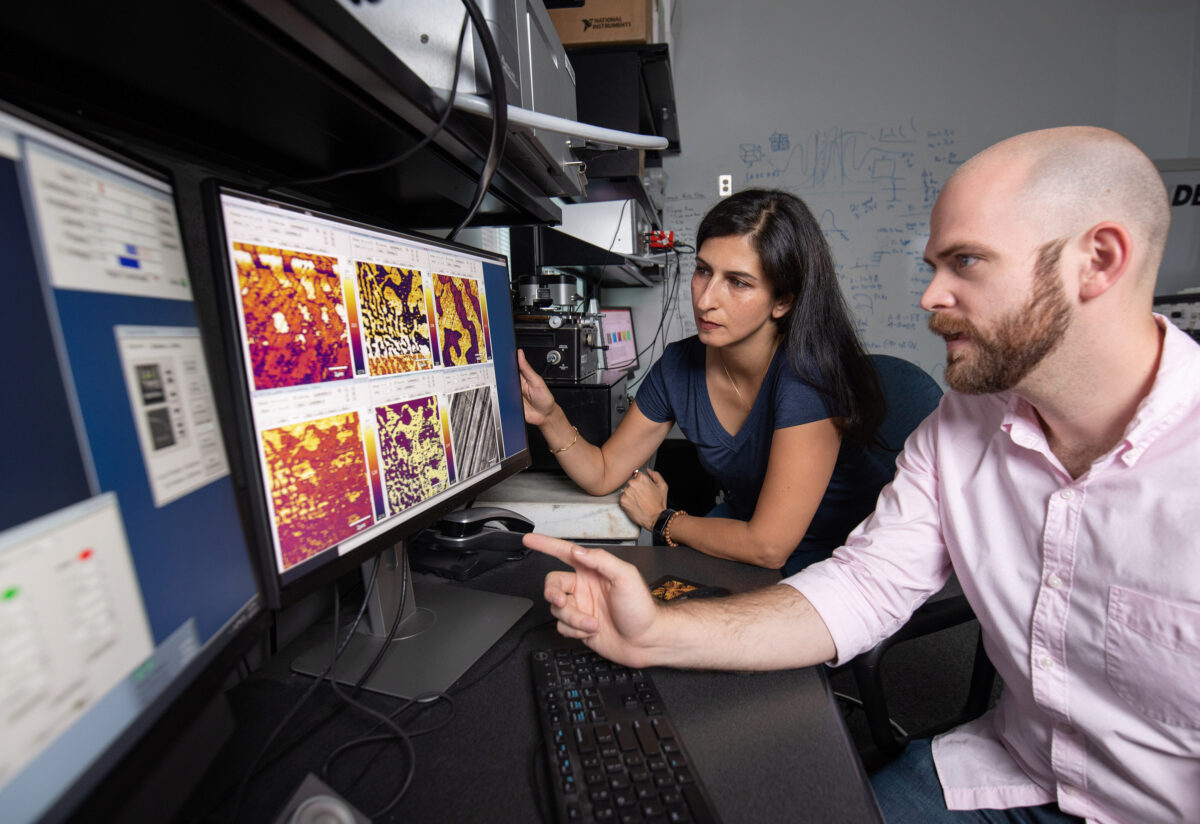
“Better, Faster, and Less Biased Machine Learning”
August 25, 2020
Machine‐learning techniques are applied more and more frequently to the analysis of complex behaviors in materials research. While significant advancements have been made over the last decade, the continuous progress in such techniques has often resulted in an increase in complexity without an increase in efficiency or interpretability.
In a recent paper published in Advanced Materials titled “Better, Faster, and Less Biased Machine Learning: Electromechanical Switching in Ferroelectric Thin Films,” Nazanin Bassiri-Gharb, Harris Saunders Jr. Chair and Professor in the George W. Woodruff School of Mechanical Engineering at the Georgia Institute of Technology discusses simple clustering and dimensional reduction (DR) analysis methods as a way to overcome some of these challenges.
Dimensional reduction approaches have been widely used to identify fundamental physical behaviors within complex datasets. These methods require less computational time and sophisticated hardware and have a lower energy cost than neural network based techniques.
If we use proper datasets, we do not need to use complex algorithms or supercomputers,” said Bassiri-Gharb. “Compared to previous work that has used neural networks and significant computational power, we used techniques that ran on a personal laptop and the analysis was completed in just a couple of hours.
In addition to the above challenge, another concern with the applicability of machine-learning techniques to materials science is the misinterpretation of data due to the results lacking a physical basis, as these methods are strictly mathematical in nature. Furthermore, any user-based, “physically reasonable” choice of results is inherently subject to bias, sometimes even leading to the “discovery” of nonphysical behaviors. As a possible solution to these challenges, dimensional stacking has been proposed previously by the same group. Dimensional stacking refers to assembly of multiple sets of data, across multiple parameters that are known to result in correlated behavior. In the most basic form, dimensional stacking can be applied to the same type of data set measured on multiple samples of the same material. In functional materials, where the functional response is modulated by one or more external stimuli, often the data are acquired as a function of such stimuli. Data sets can then be used as function of each of these external parameters, or the effects of multiple external parameters can be analyzed at the same time through stacking of the data sets expected to have physical (or chemical) correlations.
In addition to dimensional stacking, careful and critical approaches, including considering available model performance metrics, maintaining a healthy suspicion of the results, continually referring to the physical understanding in interpretation, and consideration of all selected possible outcomes are proposed as methods to minimize user bias and increase interpretability of results.
“Effectively, more complicated, more expensive or more exotic tools are not necessarily key to better results: the key remains our understanding and the proper scientific approach,” said Bassiri-Gharb.
To test the technique, Bassiri-Gharb, and co-authors Lee A. Griffin and Iaroslav Gaponenko applied easily implementable and computationally inexpensive clustering and dimensional reduction analysis methods augmented with dimensional stacking to ferroelectric switching data collected via band-excitation piezoresponse force microscopy on PbZr0.2Ti0.8O thin films. Band-excitation piezoresponse force microscopy enables exploration of the local electromechanical response of the sample at the same time as the viscoeleastic properties. According to their results, the data only supports the existence of typical ferroelectric switching with known non-ferroelectric phenomena superimposed. This stands in contrast with previous work, which applied a neural networks-based autoencoder to the same data and proposed a different interpretation based on contributions from exotic phenomena (charged domain walls in head-to-head polarization configurations).
“Machine Learning algorithms are like a black box. If you take everything that comes out without proper consideration, there is too often a chance to “discover” something that is more complicated that the actual physical phenomena. ”
Prof. Bassiri-Gharb and her team showed that through careful model parameter selection, appropriate application of the physical understanding and constraints, and knowledge of the system under investigation, one can gain better insights into the fundamental ferroelectric behavior in these materials. For example, through the application of clustering methods, the authors identified and removed from consideration localized outliers and instrumentation contributions that would otherwise compromise the results. Subsequently, leveraging dimensional stacking combined with computationally inexpensive machine-learning techniques, they derived spatially correlated piezoresponse (electromechanical) and resonance frequency (viscoelastic) behaviors. Lastly, to reduce user bias, model parameters were selected through a systematic approach based on the reduction of residual errors balanced with overfitting, ultimately resulting in a range of analyses to be considered as a whole.
“The truth is machine learning is not a panacea, nor it resolves suddenly all questions in materials science. Machine learning is never perfect, nor is the user,” said Bassiri-Gharb. “We can, as scientists, users and engineers, interpret the results but only if we use a systematic approach and open mind, without losing sight of the physical problem being handled.”
L.A.G. and I.G. contributed equally to this work. N.B.G. and L.A.G. gratefully acknowledge support from the U.S. National Science Foundation through grant # DMR‐1255379 and from the Defense Threat Reduction Agency (DTRA) through grant # HDTRA1‐15‐0035. I.G. acknowledges the support of the Division II of the Swiss National Science Foundation under project 200021_178782.