Education
- Postdoc Fellow, Harvard University, 2017-2018
- Ph.D The University of Texas at Austin, 2016
- M.S. The University of Texas at Austin, 2013
- B.S. Harbin Institute of Technology, 2011
Background
Dr. Ye Zhao is an Assistant Professor at the George Woodruff School of Mechanical Engineering and a member of the Institute for Robotics and Intelligent Machines. He is also affiliated with Decision and Control Laboratory, Machine Learning Center, and Supply Chain and Logistics Institute. He directs the Laboratory for Intelligent Decision and Autonomous Robots. He was a Postdoctoral Fellow at Harvard University, where he worked on robust trajectory optimization algorithms for manipulation problems with frictional contact behaviors. He received his Ph.D. degree in Mechanical Engineering from The University of Texas at Austin in 2016, and his dissertation work focused on robust motion planning of dynamic legged locomotion, series elastic actuation, and distributed whole-body control.
Dr. Zhao's co-authored work has been recognized as 2021 ICRA Best Paper Award Finalist in Automation, 2017 Thomson Reuters Highly Cited Paper, 2016 IEEE-RAS best whole-body control paper award finalist, and 2020 Late Breaking Results Best Poster Award, IEEE/ASME International Conference on Advanced Intelligent Mechatronics (AIM). He serves as an Associate Editor of IEEE-RAS Robotics and Automation Letters and IEEE Control Systems Letters. He was a Co-Chair of the IEEE Robotics and Automation Society (RAS) Student Activities Committee and a Committee Member of IEEE-RAS Member Activities Board. He is also an ICT Chair of the 2018 IEEE/RSJ International Conference on Intelligent Robots and Systems, and serves as an Associate Editor of IROS and Humanoids Conferences.
Research
- Automation and Mechatronics: robotics (legged locomotion and manipulation), task and motion planning, robust trajectory optimization, real-time motion planning, autonomy, and formal method based decision-making.
Automation and Mechatronics: robotics (legged locomotion and manipulation), task and motion planning, robust trajectory optimization, real-time motion planning, autonomy, and formal method based decision-making.
Dr. Zhao's research interests lie broadly in planning, control, decision-making, and learning algorithms of highly agile, contact-rich, and human-cooperative robots. Dr. Zhao is especially interested in computationally efficient optimization algorithms and formal methods for challenging robotics problems with formal guarantees on robustness, safety, autonomy, and real-time performance. The LIDAR group aims at pushing the boundary of robot autonomy, intelligent decision, robust motion planning, and symbolic planning. The long-term goal is to devise theoretical and algorithmic underpinnings for collaborative humanoid and mobile robots operating in unstructured and unpredictable environments while working alongside humans. Robotic applications primarily focus on agile bipedal and quadrupedal locomotion, manipulation, heterogeneous robot teaming, and mobile platforms for extreme environment maneuvering.
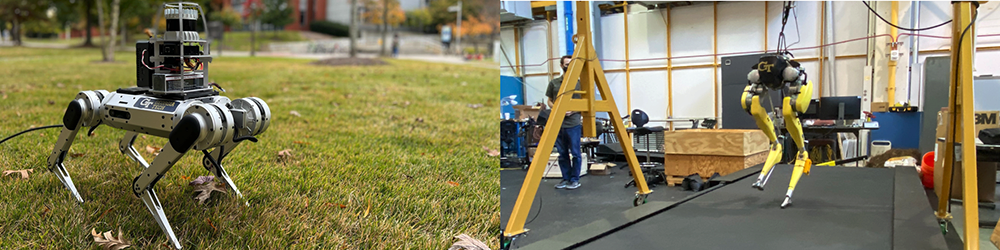
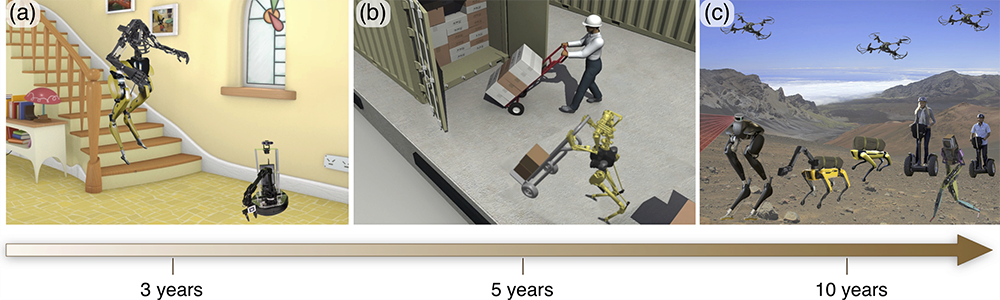
Reactive Synthesis and Decision-making of Collaborative and Agile Robots in Complex Environments
One of the main lab directions is formal methods and decision-making algorithms of dynamic terrestrial locomotion and aerial manipulation in complex and human-surrounded environments. They aim at scalable planning and decision algorithms enabling heterogeneous robot teammates to dynamically interact with unstructured environments and collaborate with humans. In particular, they are interested in robust task and motion planning approaches that (i) abstract and unify diverse, complex low-level robot dynamics generally possessing under-actuated, hybrid, and nonlinear features; (ii) computationally efficient, safe and reactive decision-making algorithms explicitly taking into account dynamic environmental events and human motions. One of our primary goals is to achieve a hierarchical and scalable planning framework with the following objectives: (i) robust, non-periodic motion planners and control barrier certificates for versatile terrestrial and aerial maneuvering; (ii) game-based reactive task planner in response to diverse and possibly adversarial environmental events; (iii) novel multi-agent decision-making approaches that decompose the entire robot team into multiple sub-teams with receding horizon approaches. They will adopt algorithmic methods at the interaction of formal methods, multi-agent systems, robust control, and machine learning. The experimental performance will be evaluated on the Buzzy Cassie robot, quadcopter, and manipulator in the lab.

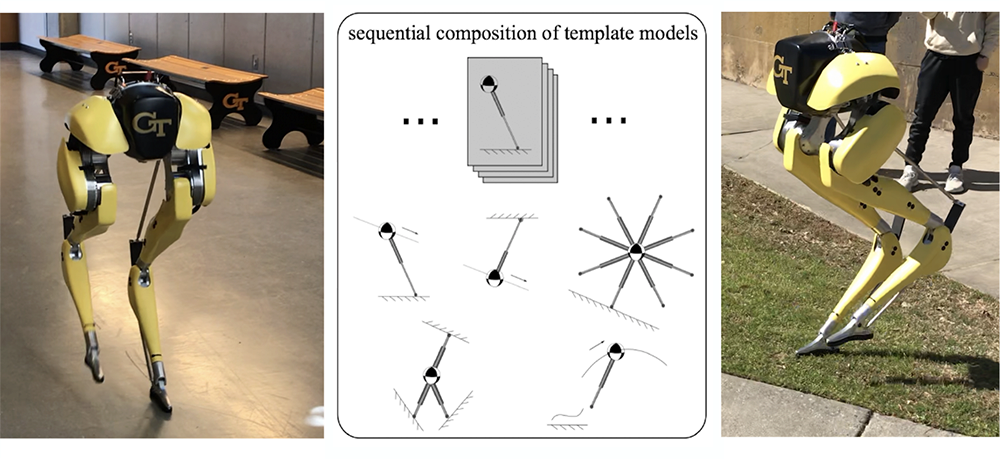
Synergizing Fast Planning and Robust Perception for Autonomous Legged Navigation
The objective of this research project is to design closed-loop perception, navigation, task and motion planners for dynamic-legged robots to autonomously navigate complex indoor environments while accomplishing specific tasks such as office disinfection. In the long-term run, a multi-agent cooperation scenario involving both quadrupedal and bipedal robots will be designed in both simulation and the mock indoor facility to validate the feasibility for real-life application scenarios. A team of legged robots will be able to autonomously navigate through various types of indoor terrain to disinfect appropriate objects while keeping humans in the loop through front-end user interfaces.
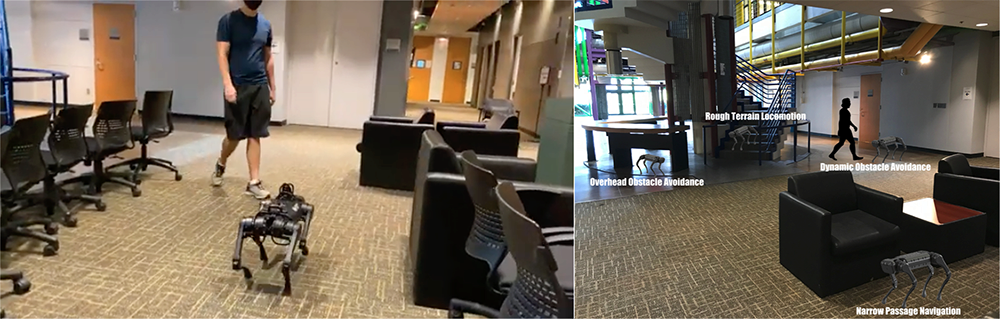
Symbolic-decision-guided Distributed Trajectory Optimization for Reactive Manipulation in Dynamic Environments
Our lab has proposed a Task and Motion Planning (TAMP) method, where symbolic decisions are embedded in the underlying trajectory optimization and exploit the discrete structure of sequential manipulation motions for high-dimensional, reactive, and versatile tasks in dynamically changing environments. At the symbolic planning level, we propose a reactive synthesis method for finite-horizon manipulation tasks via a Planning Domain Definition Language method with A* heuristics. At the trajectory optimization level, we devise a distributed trajectory optimization approach based on the Alternating Direction Method of Multipliers, suitable for solving constrained, large-scale nonlinear optimization in a distributed manner. Distinct from conventional geometric motion planner, our approach employs Differential Dynamic Programming to solve dynamics-consistent trajectories. Furthermore, instead of a hierarchical planning approach, we solve a holistically integrated optimization simultaneously involving not only low-level trajectory optimization costs and constraints but also high-level searching and discrete costs. Simulation results demonstrate time-sensitive and responsive manipulation of sorting objects on a moving conveyor belt.
Learning Generalizable Vision-Tactile Robotic Grasping Strategy for Deformable Objects
Reliable robotic grasping, especially with deformable objects such as fruits, remains a challenging task due to underactuated contact interactions with a gripper, unknown object dynamics and geometries. In this project, we propose a Transformer-based robotic grasping framework for rigid grippers that leverage tactile and visual information for safe object grasping. Specifically, the Transformer models learn physical feature embeddings with sensor feedback through performing two pre-defined explorative actions (pinching and sliding) and predict a grasping outcome through a multilayer perceptron (MLP) with a given grasping strength. Using these predictions, the gripper predicts a safe grasping strength via inference. Compared with convolutional recurrent networks (CNN), the Transformer models can capture the long-term dependencies across the image sequences and process spatial-temporal features simultaneously. We first benchmark the Transformer models on a public dataset for slip detection. Following that, we show that the Transformer models outperform a CNN+LSTM model in terms of grasping accuracy and computational efficiency. We also collect our fruit grasping dataset and conduct online grasping experiments using the proposed framework for both seen and unseen fruits.
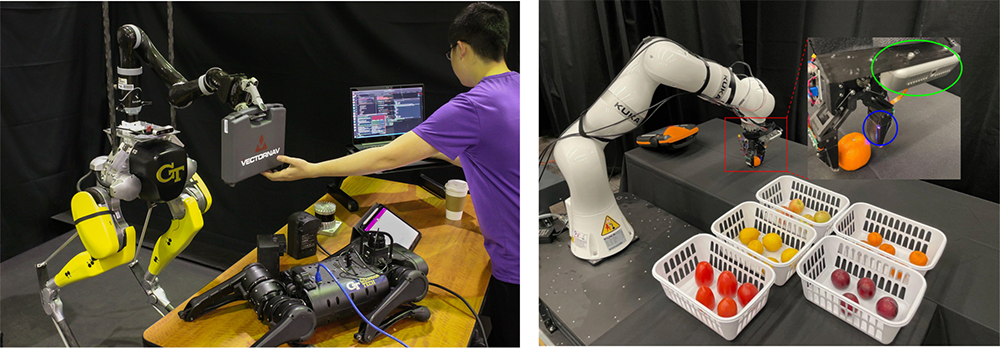
Integration of undergraduate students into experiment-focused robotics research through the VIP program.
The LIDAR Lab is currently leading a multi-disciplinary Vertically Integrated Program (VIP) team -- Agile Locomotion & Manipulation (co-advised with Prof. Seth Hutchinson, https://www.vip.gatech.edu/teams/vwt) -- that offers a transformative mechanism to engineering and science education by engaging undergraduate students in a cross-college, multi-semester, and large-scale project team. Currently, the PI leads a team of 80 undergraduate students from diverse engineering, science, and computing disciplines. In the past few years, the team won first place in the Hardware, Devices & Robotics Track of the 2021 GaTech VIP Innovation Competition and the 2020 IEEE/ASME International Conference on Advanced Intelligent Mechatronics Best Late Breaking Results Poster Award. This VIP team has been an indispensable and integrated component of our research team and actively contributed to various aspects of our experiment-focused research projects, including the design of a biomimetic upper-body manipulator and our bipedal robot Cassie foot sensor pad design and integration.
Distinctions and Awards
- IEEE/ASME Transactions on Mechatronics Technical Editor (2024 – present)
- Best Paper Award, NeurIPS Workshop on Touch Processing: A New Sensing Modality for AI (2023)
- George W. Woodruff School Faculty Research Award, Georgia Tech (2023)
- Office of Naval Research (ONR) Young Investigator Program Award (2023)
- IEEE Transactions on Robotics Associate Editor (2023 – present)
- IEEE RAS Technical Committee on Whole-Body Control Co-Chair (2022 – present)
- National Science Foundation CAREER Award (2022)
- IEEE Control Systems Letters Associate Editor (2022-present)
- First place in the Robotics Track of the VIP Innovation Competition, Georgia Tech (2022)
- IEEE Senior Member (2022-present)
- Best Automation Paper Award (Finalist), IEEE International Conference on Robotics and Automation (ICRA 2021)
- First place in the Hardware, Devices & Robotics Track of the VIP Innovation Competition, Georgia Tech (2021)
- Woodruff Academic Leadership Fellow, Georgia Tech (2021)
- Late Breaking Results Best Poster Award, IEEE/ASME International Conference on Advanced Intelligent Mechatronics (AIM 2020)
- Class of 1969 Teaching Fellow, Georgia Tech (2020)
- “Thank a Teacher” Certificate, Georgia Tech Center for Enhancement of Teaching and Learning (2019-2021)
- IEEE Robotics and Automation Letters Associate Editor (2019-present)
- Woodruff School Teaching Fellow, Georgia Tech (2019)
- Thomson Reuters Highly Cited Paper (2017)
- IEEE-RAS Best Whole-Body-Control Paper Award Finalist (2016)