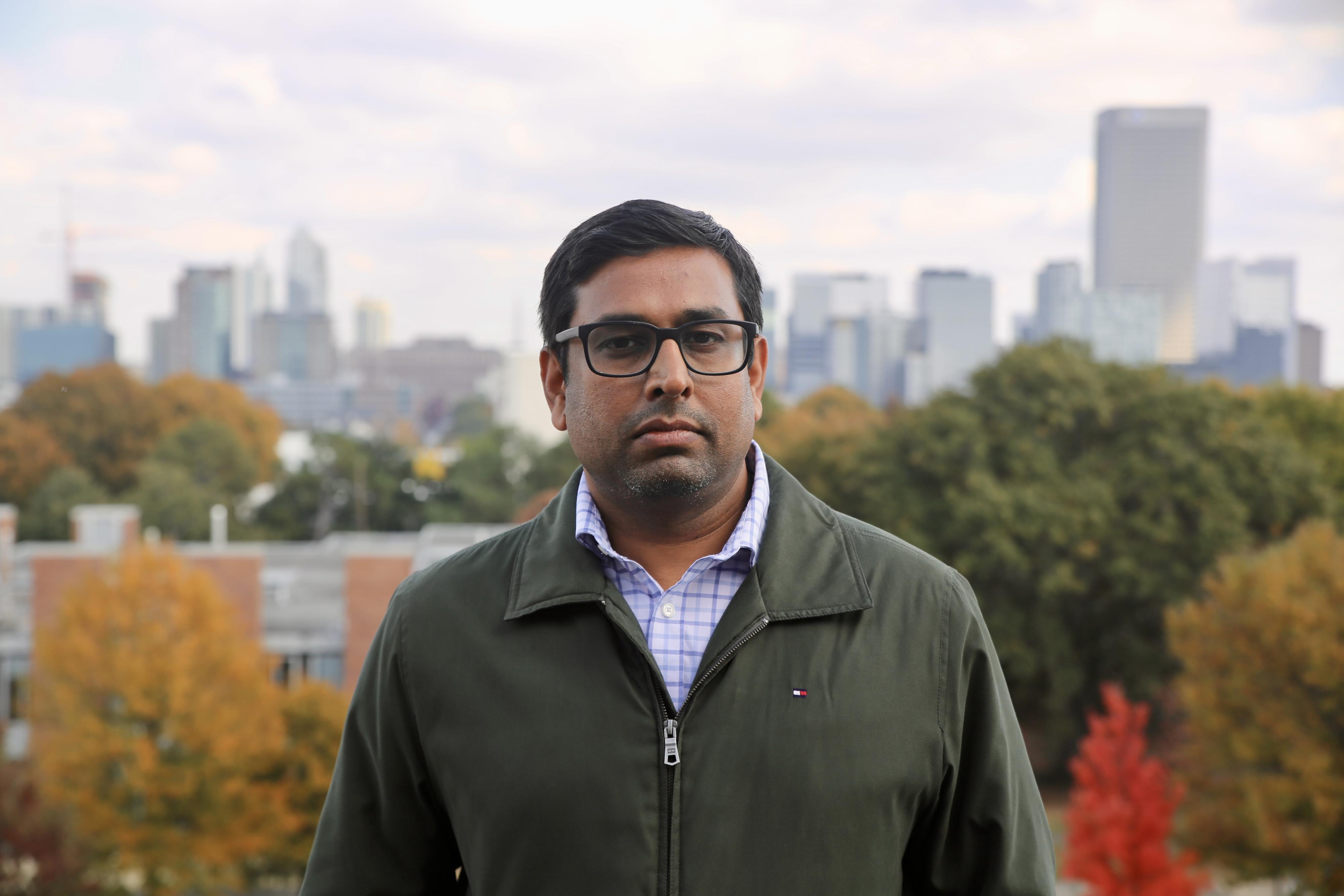
Research Team Awarded $7.3 Million for Machine Learning Assisted Development of High-Fidelity Two-Phase Models
October 25, 2024
A collaborative team from Georgia Tech, Purdue University, Case Western Reserve University (CWRU), Michigan State University (MSU), and Brown University have been awarded a combined $7.3 million from the Office of Naval Research (ONR) as part of the Multidisciplinary University Research Initiative (MURI) program. MURI seeks to fund research teams with creative and diverse solutions to complex problems and is a major part of the Department of Defense’s (DoD) research portfolio.
Satish Kumar, Frank H. Neely Professor in the George W. Woodruff School of Mechanical Engineering, along with his collaborators, received the five-year award for their project, Machine learning Enabled Two-pHase flow metrologies, models, and Optimized DesignS (METHODS). Georgia Tech, CWRU, and Brown University will lead efforts of machine learning (ML)-assisted discovery of mechanistic two-phase models and generalized two phase flow model development. Purdue University (lead on the project) and MSU will lead efforts in performing two-phase flow experiments and generating high resolution data for the project.
This interdisciplinary project will develop an end-to-end framework comprising metrology development, data interpretation, model discovery, training, and uncertainty quantification, as well as generalized approaches for predictive design and control of two-phase flows. This research integrates cross-cutting ML approaches, applied in a novel manner to two-phase flows, to enable transformative advancements in measurement, modeling, and design capabilities.
The designers of two-phase flow systems end up using empirical correlations for ease of use and lack of high fidelity two-phase models as they have historically been developed and validated using a few globally measured parameters. To address this gap and provide a long-sought generalized modeling framework for prediction of two-phase flow and heat transfer, the team proposes a multidisciplinary convergence among available liquid-vapor phase change transport modeling domain knowledge, advanced fluid physics metrology, and emerging physics-informed machine learning and computer vision techniques.
The team's focus is centered around interrogation of flow boiling and condensation in channels as the fundamental model of a majority of the two-phase flow scenarios in components and systems of interest.
Access to generalized predictions of two-phase flows across all operating conditions and transients will benefit realistic systems that have many interacting heat sources and sink with substantially reduced design cycle time.
“Impact will be significant for dual-use commercial automotive, aerospace, computing, and other applications where two-phase cooling is sought,” said Kumar.
METHODS brings together experts in two-phase flow heat transfer, advanced metrology for fluid flows, and applied ML and machine vision. In addition to Kumar, the team includes Professor Justin Weibel (lead PI) and Professor Fengqing Zhu from Purdue University; Professor Minami Yoda and Professor Manoochehr Koochesfahani from MSU; Professor Chirag Kharangate from CWRU; and Professor George Karniadakis from Brown University.