Education
- Ph.D. Massachusetts Institute of Technology, 2015
- M.S. Massachusetts Institute of Technology, 2010
- B.S. Massachusetts Institute of Technology, 2008
Background
Dr. Ellen Yi Chen Mazumdar joined the Woodruff School of Mechanical Engineering at Georgia Tech in 2019. Her research group currently focuses on developing new sensor systems and diagnostic techniques for robotic, biomedical, combustion, fluid, and hypersonic applications. Her group studies these physical phenomena using interdisciplinary tools including magnetostatics, spectroscopy, ultra-fast laser optics, digital holography, stochastic system identification, machine learning, and mechatronics. Her previous experience includes a postdoctoral research position at the Engineering Sciences Center at Sandia National Laboratories in Albuquerque, NM.
Research
Scientific advancements in fields ranging from flow diagnostics to autonomous vehicles rely on the ability to quantitatively sense, measure, and understand physical phenomena. As we study more complex systems in harsher environments, new methods are needed for gathering and perceiving information.
In order to understand the underlying physical processes in hypersonic, explosive, combustion, multi-phase flow environments, a variety of new diagnostic techniques need to be developed and employed in test-bed systems. Currently, the lab is exploring variations on optical diagnostic techniques including digital in-line holography, phase-conjugate mirrors, time-of-flight Kerr gating, imaging pyrometry, laser-induced incandescence, and other ultra-fast methods. In addition to optical approaches, new sensing methods using magnetic fields are also being studied for making temperature, vibration, and fluid vorticity measurements remotely.
Sensing is also a key component to many diverse systems and contributions to sensing can revolutionize the fields of autonomy, controls, and robotics. For systems like continuum robotic endoscopes and portable imaging spectrometers, new sensors, actuators, and control architectures are needed. By combining new sensor designs with encoding concepts, compressive sensing, and machine learning methods, Dr. Mazumdar’s lab seeks to improve perception and performance for complex human-machine systems.
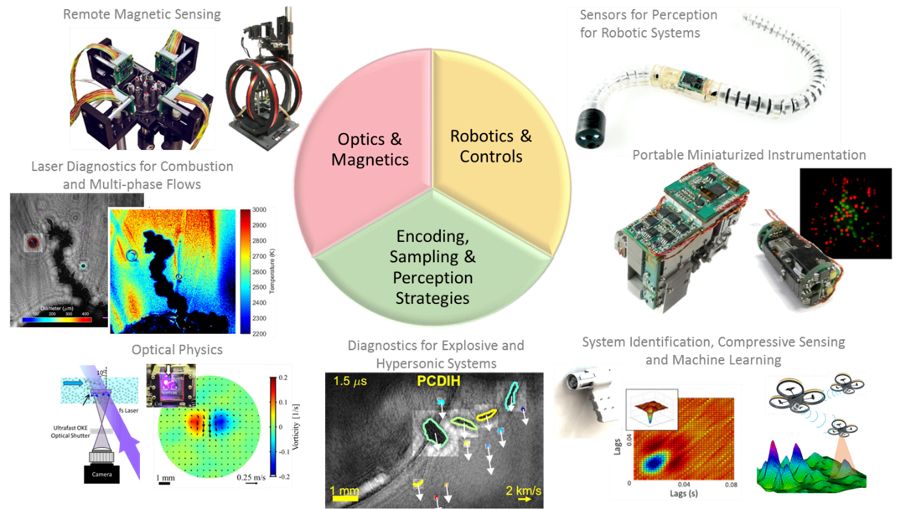
Figure 1: A multi-disciplinary approach to instrumentation, sensing and diagnostics.